The most common data hygiene problems and how to solve them
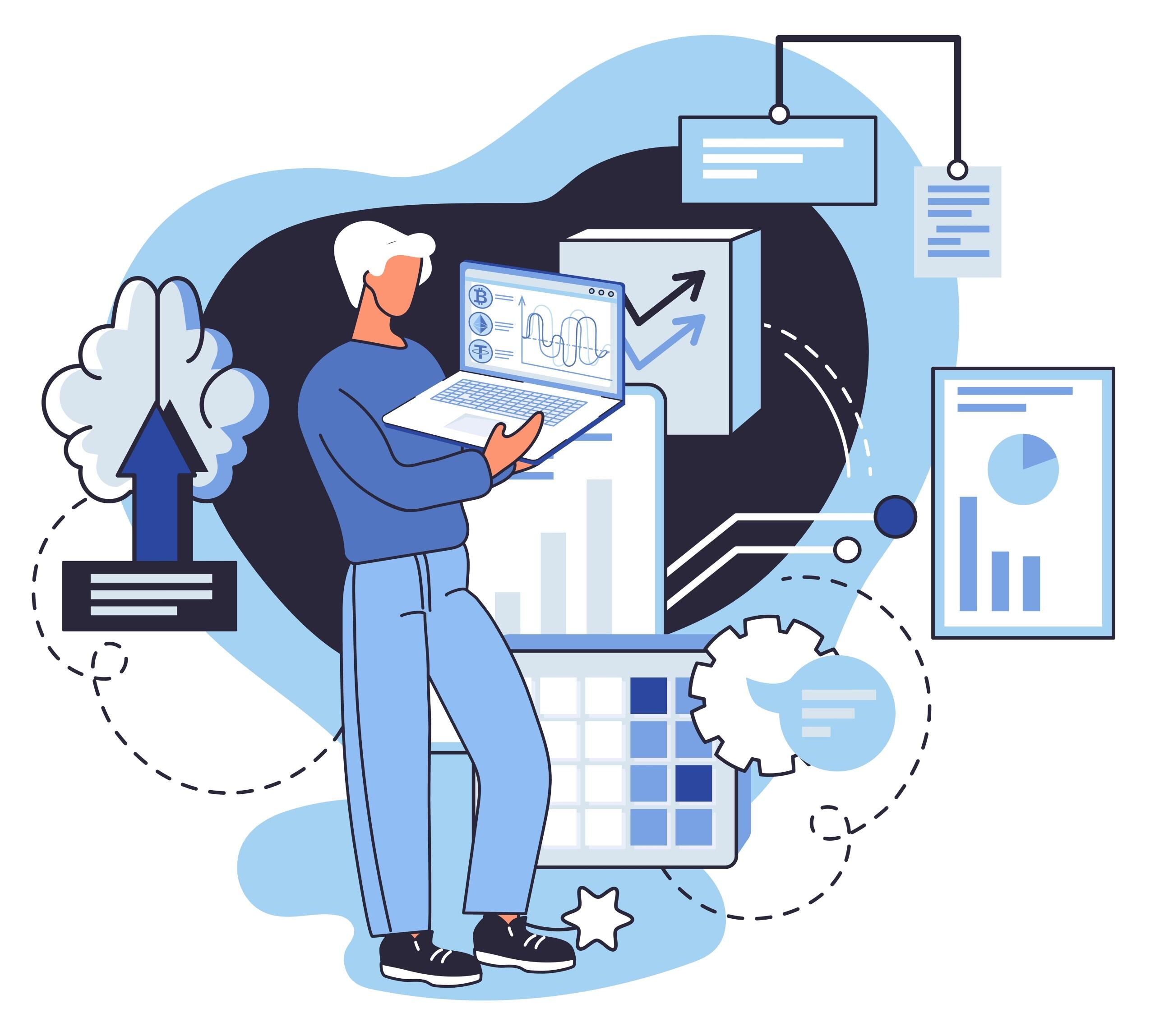
Inaccurate data makes it difficult to respond to market changes, according to 77% of respondents in Experian’s 2022 survey. Meanwhile, many companies struggle to take care of the quality of their data. Managers indicated that poor data quality negatively impacts the customer experience (39%) and 84% highlighted employees’ lack of data analysis skills.
From this blog post you will learn:
- what data hygiene is
- what the main data problems are
- what causes data problems in companies
- what makes data hygiene difficult.
What is data hygiene?
Data hygiene is the process of managing, storing, updating and deleting data. Data must be up to date, correct, complete and in line with the requirements of the organization in order for modern technologies to use it. Therefore, data hygiene becomes an indispensable part of management. Regular data monitoring and risk assessment help to maintain data quality. And this translates into better business decisions and minimises the risk of data breaches.
Read our article: Clean data, clear path: mastering data hygiene in your organization.
The Salesforce portal found that 73% of leaders believe that reliable data supports sound decision-making. Meanwhile, many companies struggle to maintain the quality of their data. According to a study published by Harvard Business Review, on average 47% of new data records contain at least one critical (i.e. job-impacting) error. While a result of 3% is considered ‘acceptable’ – and this is at the lowest possible standards.
It is this low data quality that causes problems when either integrating or automating data. According to the publication Journal of Industrial Engineering and Management ‘The costs of poor data quality’, 88% of data integration projects fail or go significantly over budget. The reason? Poor data quality. Additionally, the consequences of a data breach can negatively affect the rights and freedom of individuals.
Typical data problems are most often:
- Duplication: records in the database appear more than once. Sometimes the same person, company or location appears in the database several times, but with a different set of data.
- Data missing: the search does not show all the data required in a specific situation. Thus, there is no complete picture of the situation.
- Inconsistency: the same data exists in different formats in several tables. You then create several files, each containing different information about the same object or person.
- Inaccuracy: there are incorrect or outdated values in the database. It is then difficult to make informed and optimal decisions.
Poor data quality – examples of problems in a company
Sales and marketing
DiscoverOrg conducted a study on data quality in companies. It showed that sales and marketing departments lose around 550 hours and up to USD 32,000 per sales representative due to the use of incorrect data.
According to the MIT Sloan report, data analysts spend 60% of their working time cleaning and organizing data. Other employees waste up to 50% of their time manually sifting through important data and improving its quality.
In marketing, this can generate unnecessary expenditure. In turn, potential customers will be annoyed when they receive the same content several times because of duplicate data. And this is a fairly common problem when there are several records in a database with the same name but stored differently. A minor mistake that causes considerable image damage.
In online sales, a customer may receive the wrong product through poor quality data. This is the risk when there is no reliable data about the products and the target audience. And what if there is no automatic record verification in the database? And what if the customer’s VAT number is accidentally included in the telephone number field? The courier will certainly not get through to the customer with delivery information.
Finance and banking
In financial reporting, the consequence of inconsistent data is multiple answers to the same question. Inaccurate reports are produced that are misleading. They can give a false sense of security or just the opposite: a worrying sense of financial insecurity.
Incorrect revenue or cost data can lead to a misallocation of resources or an overly optimistic assessment of the profitability of a new project. Differences in accounting methods or cost classification result in inconsistent financial reports. This makes it difficult to assess company performance and make strategic decisions.
Manufacturing
Production is also sensitive to data quality. Seemingly minor inaccuracies in data often cause losses and lead to wrong decisions. For example, outdated material prices in a cost estimate can distort margins.
Poor data quality negatively affects production growth and profits. The Institute of Industrial Management at RWTH Aachen University has shown that the supply chain loses between 1% and 3% of productivity due to data quality problems. This costs manufacturers an average of 0.5% of their revenue. The quality of data aggregated by companies has an impact on market success and stable growth.
Manufacturing data is often complex. It can come from multiple sources, including machines, sensors and software systems. Integrating data from different sources can be difficult and require a significant commitment of resources. This situation makes data analysis difficult. Although manufacturing companies implement MES-class systems, this does not solve the problems of deeper data analysis.
Companies then still face the challenges of analyzing huge volumes of data. This data flows in daily and must be properly translated into the individual areas of the production company. For example, into the personal goals of employees. Added to this are the constraints of being able to change parameters or produce additional reports or visualisations. Each change leads to additional costs, not to mention increased time to obtain data or produce reports.
According to the report prepared by Deloitte has prepared the report ‘2024 Manufacturing Industry Outlook’. As many as 45% of decision-makers from manufacturing companies expect to further increase operational efficiency by investing in the Internet of things (IoT).
This technology connects the product, the end user and the manufacturer. The goal? The manufacturer gathers information about how the product is used and its performance. The manufacturing company gains access to more data that it can use in various ways, e.g. in designing new products, repairing products under warranty. However, it needs to be able to interface this data with other systems.
Supply chain
It is very difficult to automate supply chain processes if decisions are based on unreliable location information. It is also unclear what data should be used to make decisions. It is difficult to control inventories and plan orders when data is outdated, incomplete or wrong.
Incomplete or heterogeneous product data can make it difficult to identify and track products. The consequence will be delays in deliveries, but also difficulties in meeting regulatory requirements. It follows that product tracking is increasingly important in light of environmental, recycling and circular economy regulations.
Management
High-quality data improves a company’s ability to achieve long-term goals. If data quality is poor, it can:
- adversely affect the ability to adapt and respond quickly to new trends and market conditions;
- increase the difficulty of complying with requirements that arise from key privacy and data protection regulations such as GDPR, HIPAA and CCPA, and sustainability (ESG);
- make it more difficult to use predictive analytics on company data, which may make decisions riskier;
- make it impossible to prevent, for example, machine breakdowns through earlier maintenance. This increases downtime and reduces productivity.
What hinders data hygiene?
The growing diversity of data sources
For many years companies used only data generated by their own business systems. Data ‘silos’ were the common standard: separate ones for sales and production or marketing.
Nowadays, businesses draw data from a variety of sources: the Internet, the Internet of Things, scientific publications, experimental results, etc. The more sources there are, the more difficult it is to control the quality of the data and to ensure that it is not altered or modified.
Every additional system added to the data processing engine increases the risk of losing the value of this data. It is more likely to change or disappear because different sources generate different types of data.
This is especially true of unstructured data, i.e., data not organized according to a defined data model. It is estimated that unstructured data now accounts for around 80% of all data worldwide. Any processing of such data is risky.
Increasing amount of data
We live and operate in the era of big data. The amount of data has doubled every three years since 1970 and is constantly increasing. The more data there is, the more difficult it is to collect, clean, integrate and achieve a reasonably high quality. On top of this, the time taken to process them is increasing, and the processing itself is an increasingly complex. It is worth noting that data overload and the associated stress can negatively affect the mental health of employees.
Accelerating the speed of data use
‘Real-time data’ has become a fashionable buzzword over the past five years. The more data you generate, the faster you need to process it. Unfortunately, when you increase the speed, you risk clogging up your systems.
Data is like liquid in a pipe: the faster it flows, the more likely the pipe is to burst. So, the only solution is to widen the ‘data pipe’. This will ensure that data is processed at the same speed at which it comes in. This is a difficult task and is worth doing in collaboration with experienced experts.
However, real-time data processing is still a relatively new field. This means that some important data may be missed and irrelevant data be successfully processed. This is why it is so important to regularly monitor the data and ensure its hygiene.
Lack of own data quality standards
Product quality standards have existed since 1987, when the International Organization for Standardization (ISO) published ISO 9000, while data quality standards have only existed since 2011 (since ISO 8000). They are still being developed and there is no single universal standard. Universal ways and procedures that can be successfully applied to every company are still lacking. A company must therefore develop its own rules. It is worth using the support of experienced consultants and data analysts.
You can find out about data hygiene best practices in our article: Get more out of your data – data hygiene best practices
Data hygiene has a significant impact on the efficiency, health and safety of an organisation. Data from outdated or erroneous sources can lead to wrong decisions that can have a negative impact on the organisation.